Reviewing 1500 resumes in 6 hours with AI-Assisted Application Review in Ashby
8 minute read
Throughout my two decades in Talent at various companies of different sizes, managing application volume has always been a significant challenge—getting through the high numbers, maintaining consistency across reviewers, and the risk of missing out on candidates in a large pool. The stakes are high.
That’s why I was thrilled to work closely with our product team over the past couple months, putting our new AI-Assisted Application Review feature through rigorous testing and evaluating it with my 20+ years of experience in this crucial part of the hiring process.
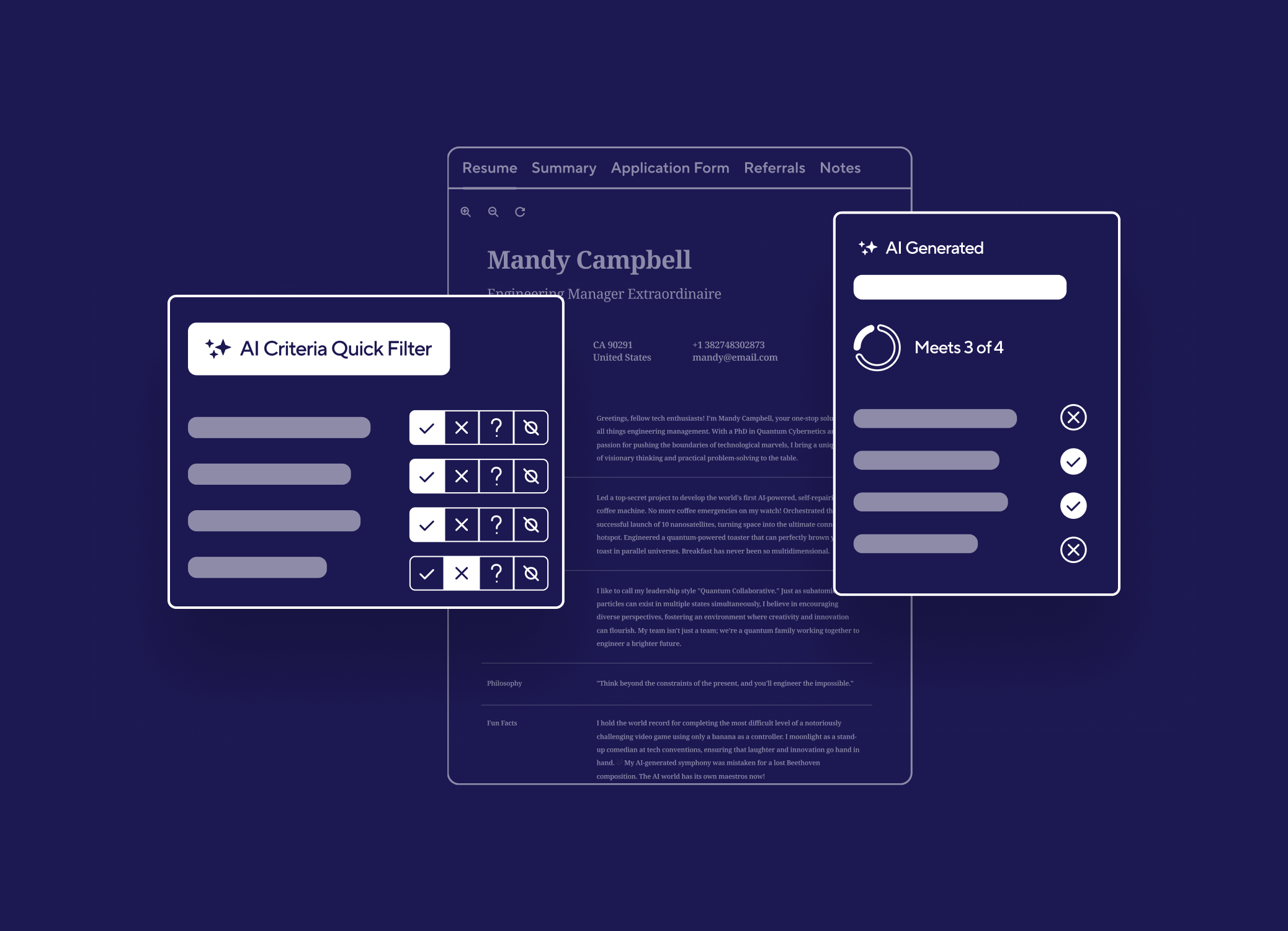
It’s no overstatement to say this new tool has transformed the way I approach this work, combining efficiency with a thoughtful and responsible deployment of AI technology.
In this post, I’ll walk through what excites me the most, how it changes the shape of the work, and the impact on candidates and talent teams.
The problem with manual resume review (old way)
Traditionally, with chronological application review, you process candidates in the order they apply. You might use location filters or booleans, but for the most part, you reject or advance candidates linearly, working through the numbers.
Some of the best dedicated screeners are expected to review 2,000 applications per week.
The challenge with this approach has always been that you never know what type of resume or application is coming next. A good one. A bad one. The perfect match.
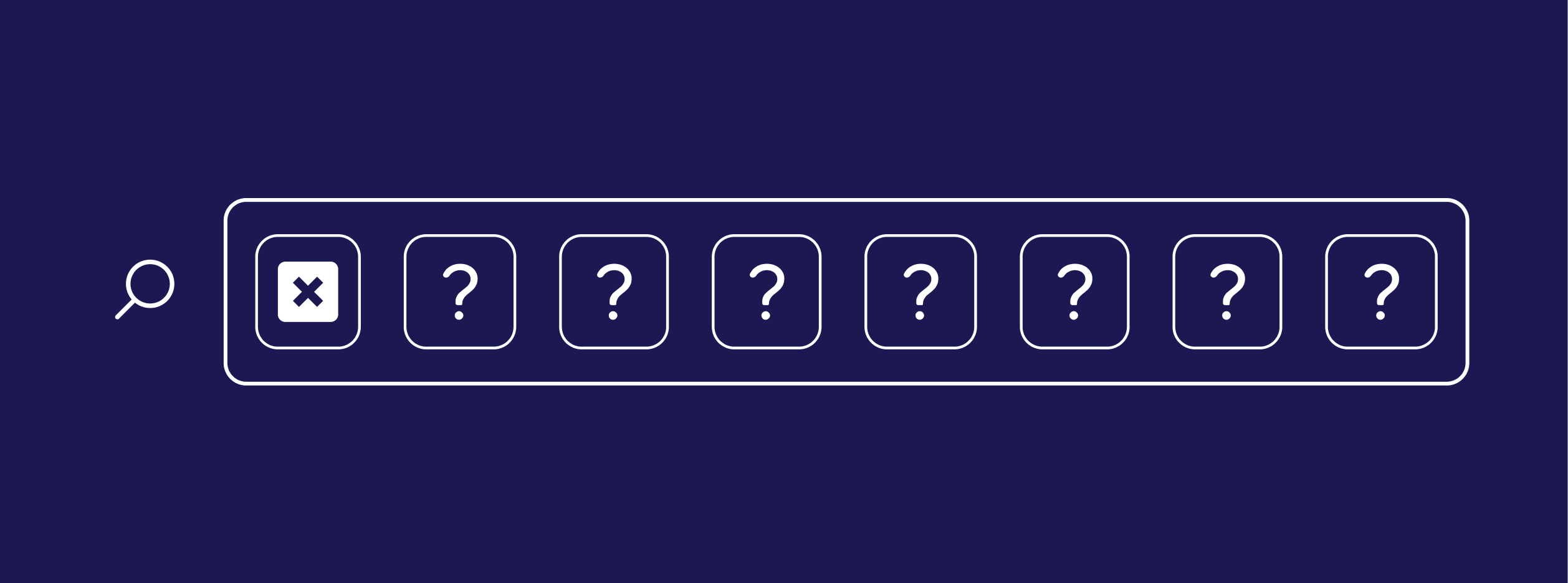
When you don’t know what’s coming, your brain has to reset with each new resume. You need to mentally juggle the minimum requirements, nice-to-haves, location details, and more before making a reject/advance decision.
Then, you do it all over again for the next resume.
Repeating this process hundreds of times across multiple jobs turns what could be a strong signal for your pipeline into a significant time drain.
When inbound volume is high and there’s always more to do, humans are more likely to take shortcuts, knowingly or not. As the numbers increase, people tend to lose focus and settle into “autopilot” review mode.
They might only glance at job titles or schools. Biases inevitably creep in as humans try to work more efficiently.
You might end up reviewing just 10-15% of applications, leaving the rest untouched, which introduces a real risk of missing out on great candidates.
Larger applicant pools often require multiple reviewers, leading to inconsistencies in evaluations.
When the volume reaches a certain threshold, it becomes an unfair and nearly impossible task.
There have been ways to address this in the past, but none compare to the step-change I’ve experienced with AI-assisted Application Review.
A new way of working
So how did I accurately and confidently review 1,500 resumes in 6 hours? By changing the mental model and approach with the help of our new feature.
Everything leading up stays the same — I learn the job description inside and out and qualify the role. But now, using Ashby's AI-Assisted Application Review feature, I document a series of specific criteria that applicants should meet.
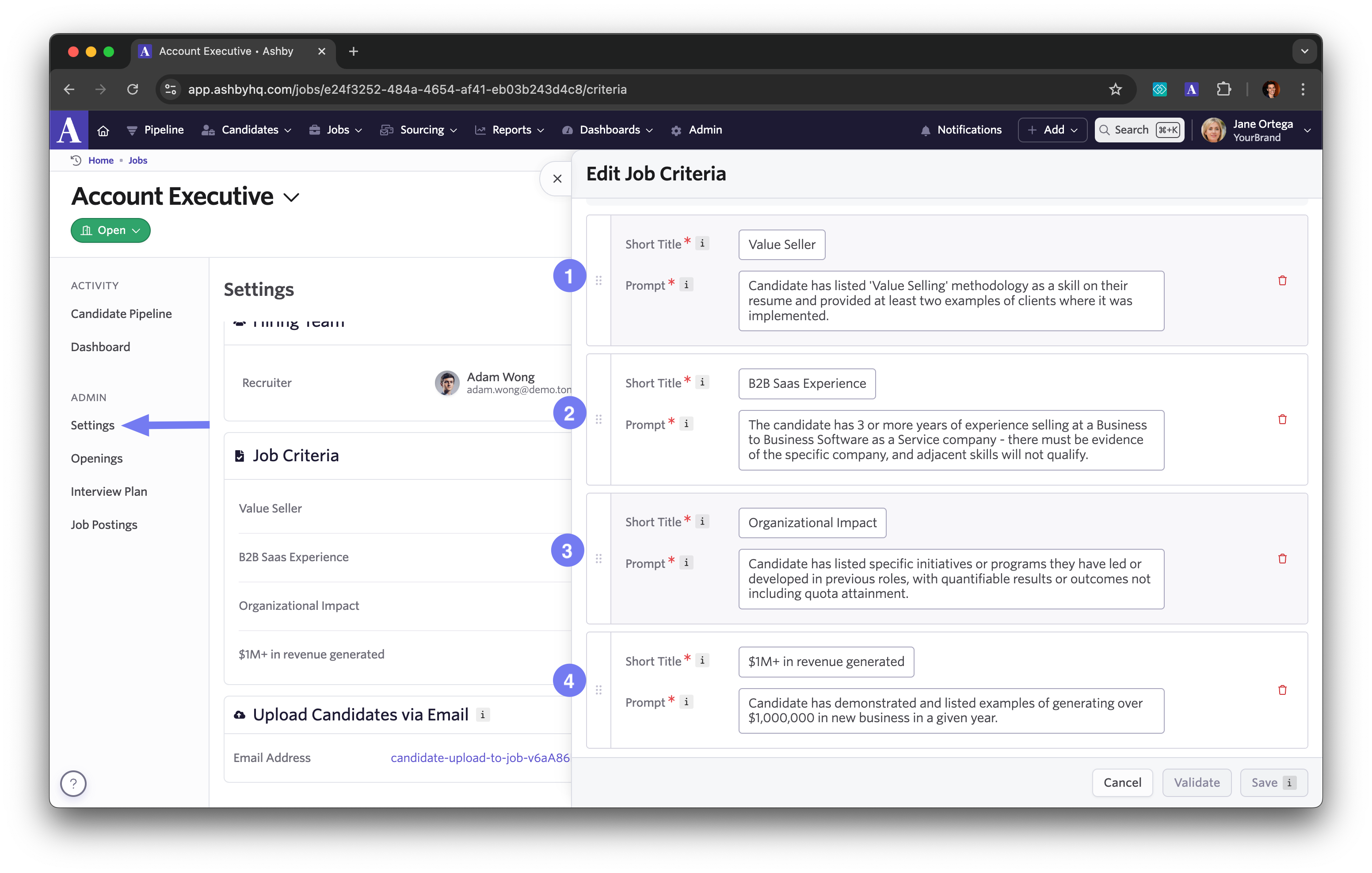
This criteria uses AI to analyze all of the resumes and informs me, as the reviewer, whether they meet my defined criteria.
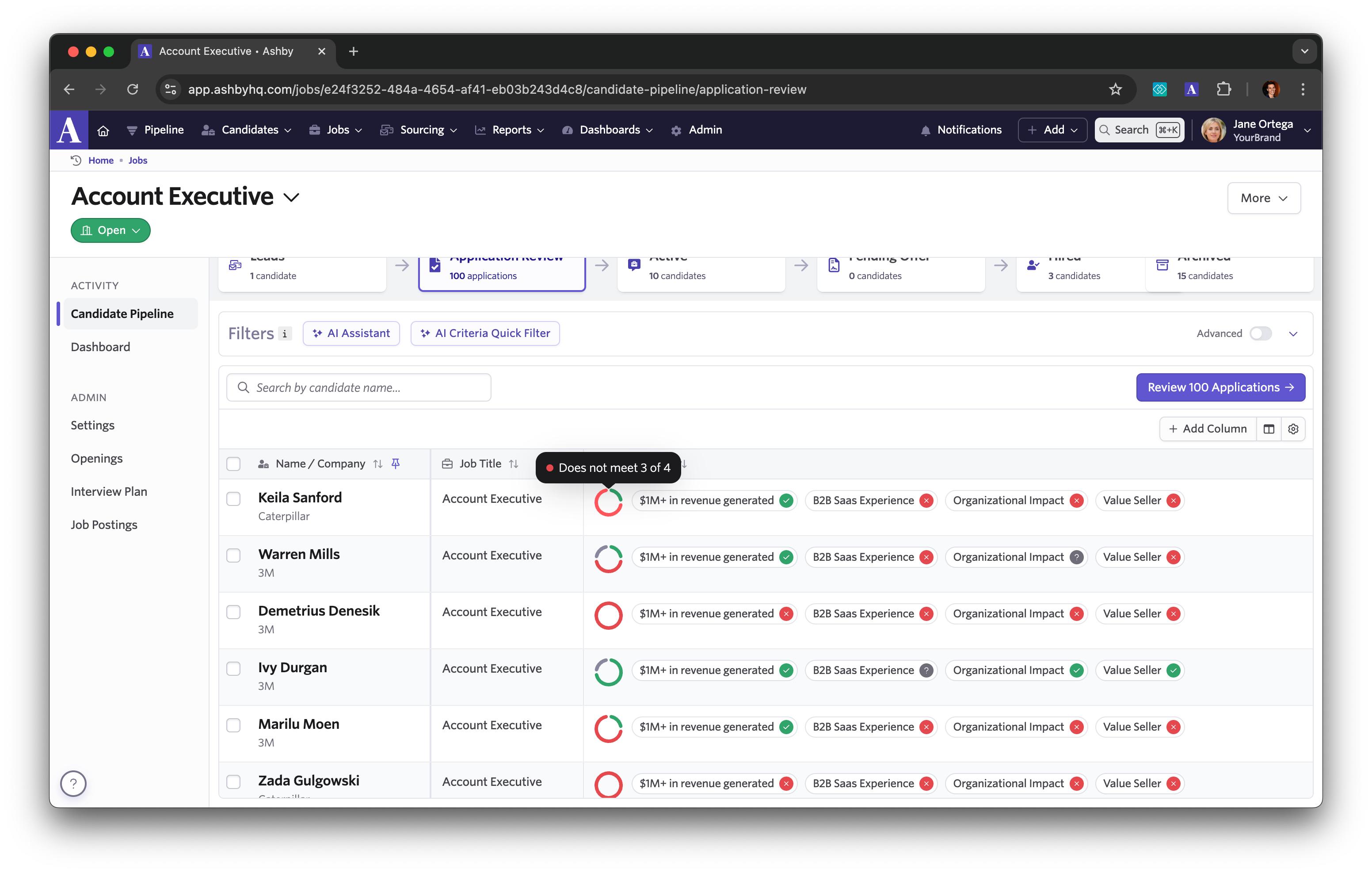
No decisions are made for me. No scoring or ranking is involved. The AI analyzes the resume and produces a “Meets” or “Does not Meet” for each criterion I’ve set. Additionally, I have the advantage of documenting specific and objective criteria directly within the ATS.
Now, when I enter the review process, I can group similar candidates and, most importantly, verify applications based on criteria matching.
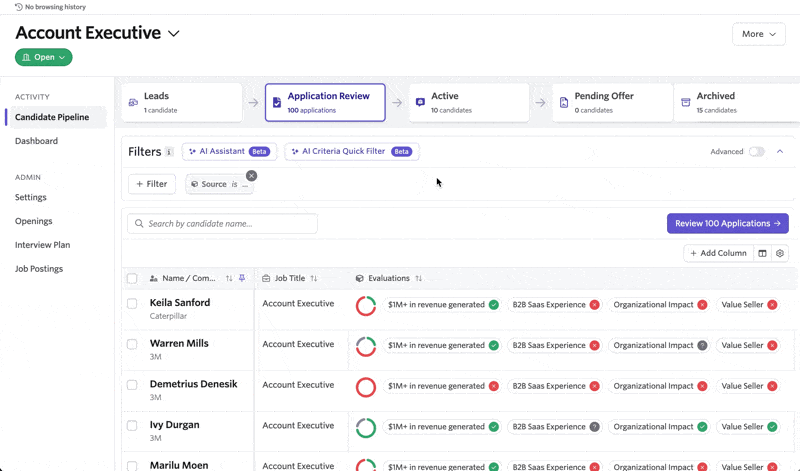
This is where I experience a step-change in efficiency. I can approach each batch of applications with the same context. When reviewing a batch of applications that all meet my predefined criteria, I can move faster by verifying the AI-assisted analysis of the resume.
No more reloading context and starting from scratch each time I review the next resume.
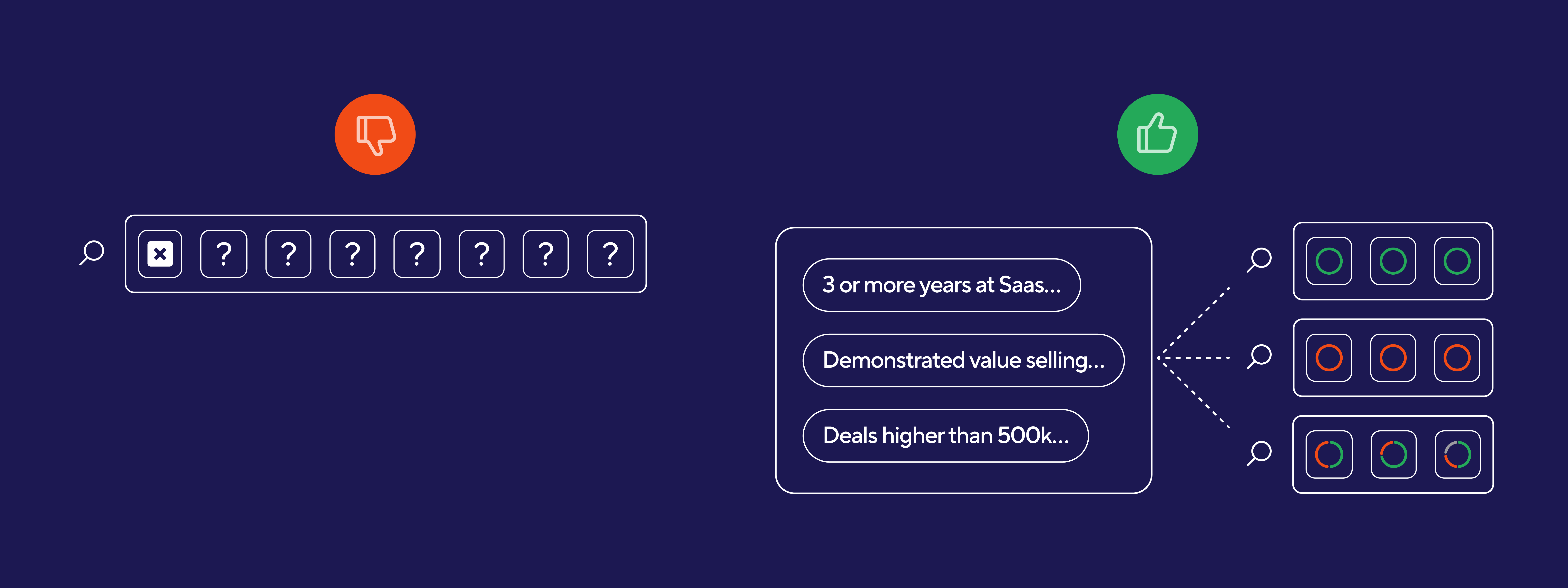
Using the most advanced LLMs offers access to a vast library of knowledge on demand during the review. The phrase “2 years B2B SaaS experience” is surprisingly tricky to verify quickly, but the AI does an excellent job of providing a clear signal along with an explanation of its reasoning, which can serve as a helpful starting point.
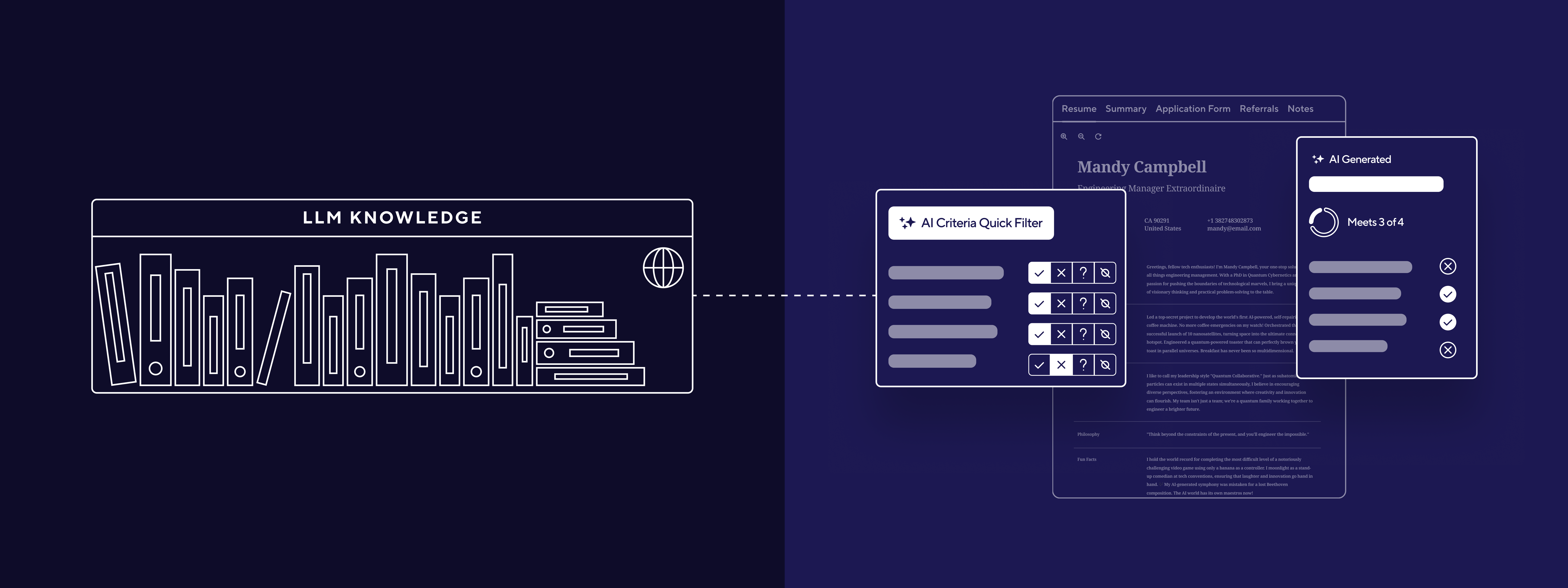
We can rely on AI assistance for otherwise time-consuming tasks, multiplied over hundreds of resumes.
For a full walkthrough on how this feature works, check out this article: AI-Assisted Application Review.
What does this mean for talent teams?
Will this replace roles and responsibilities on talent teams? No. Will teams need to grow as fast? Probably not. As I mentioned earlier, at its core, this is a massive time-savings tool. It expands the capacity of the team you already have in place.
The second-order effect of this means you can reallocate time to higher-touch sourcing strategies. You’re also able to more accurately review applicant volume, allowing more talent to be identified through your inbound channel.
You can document specific criteria and validate these with hiring managers, strengthening your partnership and collaboration along the way.
This naturally creates a feedback loop with the quality of the job description. If unsuitable talent is coming through, it signals the need to refine the criteria and improve the job description to reflect that.
Understandably, adopting this technology into your process comes with a healthy dose of “What about...?” Whether it's regulation, introducing biases, or in-product controls, there’s a lot to consider. To help talent teams embrace this kind of advanced technology, we’ve given as much thought to addressing these concerns as we have to the functionality of the feature.
To see the full picture of everything we’re doing to prevent bias, comply with regulations, and provide peace of mind for your team when using this feature, head over to the product blog post.
What does this mean for candidates?
The job of the candidate remains the same as it has always been in the application process: clearly communicate, through your resume and application, how you meet the minimum requirements in the job description.
The same reviewer actions—advancing or rejecting—are being taken. The AI assistance serves as an input to the decision-making process, not a replacement. Ultimately, talent acquisition teams are responsible for finding the best talent, and any shortcuts or blind advancing/rejecting run directly counter to that mission.
When used in the way we built this feature, AI assistance reduces biases by focusing on objective, specific, and consistent criteria. It also levels the playing field for job seekers who may not have a referral or direct connection to the company they aspire to work for.
What does this mean for the future?
We covered a lot of topics in this post, and here are some key takeaways that will have an immediate impact on your organization:
- The time saved during application reviews allows you to refocus and reallocate efforts to higher-touch activities in your recruiting process.
- AI assistance depends on reviewer decision-making. No action is taken for you, and it remains the reviewer’s responsibility to approve or reject.
- Specific, objective criteria reduce bias. Reviewers across large teams work from the same criteria analysis, eliminating the need for implicit shortcuts to handle high volumes.
- Talent teams and hiring managers strengthen their working relationships by collaborating on creating the best job criteria, helping with calibration and downstream alignment.
But where are we headed from here?
Over time when adopting this tool I suspect inbound applications to be a far more valuable channel for talent teams. Teams can surface and quickly action top talent in their applicant pool leading to better inbound outcomes.
Secondly, while the time savings from AI-assisted application reviews allow you to focus more on sourcing strategies, you may also find sourcing costs decreasing as inbound yields more hires.
Properly defining criteria and processing applications introduces greater objectivity into the screening process. This creates a significant opportunity to monitor and improve your Quality of Hire. Drawing a direct line between criteria setting and Quality of Hire is something I’m particularly excited about, and it's made possible entirely through Ashby reporting.
Finally, we’re in a place where much of the future is uncertain. In this uncertainty, it’s crucial that we continue to deliver on our AI product philosophy pillars and provide meaningful value to meet our customer’s evolving needs.
AI-Assisted Application Review is our approach to responsibly introducing the most advanced AI technologies so that our customers can navigate the continually changing talent acquisition landscape.